Tom Broeg
My name is Tom Broeg and I’m currently working as a researcher in the “Thünen Earth Observation (ThEO)” group, located at the Thünen-Institue of Farm Economics. With our Project “KlimaFern” we are combining methods of remote sensing and digital soil mapping to improve the reportability of carbon emission in the agricultural sector. My work is focused on digital soil mapping and soil science, especially in the context of soil organic matter. In cooperation with the Thünen-Institue and the University of Thübingen, I’m currently working on my Ph.D. and the development of a remote sensing-based soil monitoring system.
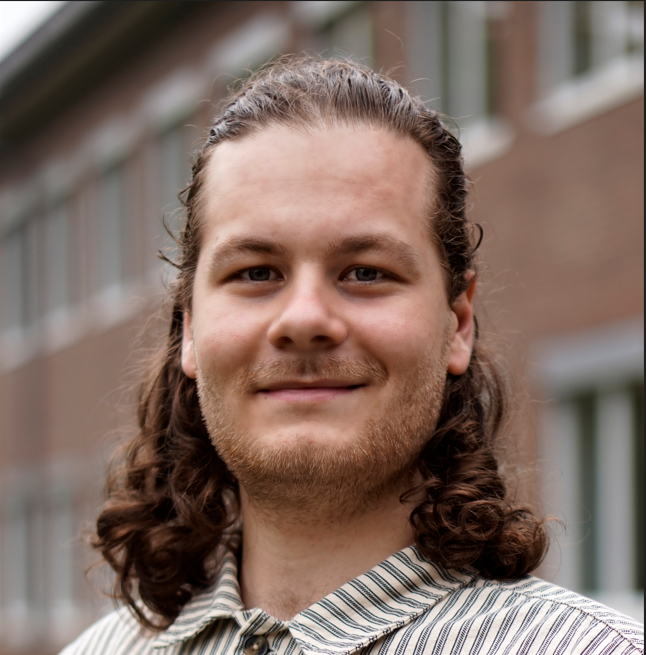
Sessions
The monitoring of soil organic carbon (SOC) in cropland is crucial, as soil health plays an important role in ensuring sustainable agricultural productivity and in reducing carbon emissions. Remote sensing technologies offer a promising opportunity to monitor soil properties on a large scale. Bare soil maps can be derived from satellite images and used to estimate soil properties, like the SOC content, based on spectral properties. However, analyzing such data requires processing large amounts of information from different sensors and time frames, which can be challenging. The Framework for Operational Radiometric Correction for Environmental Monitoring (FORCE) offers an interface to handle and analyze satellite data cubes and has been successfully used to create analysis-ready data in numerous studies. This work focuses on the generation of soil reflectance composites based on the FORCE data cube and the construction of a bare soil data cube to estimate trends of dynamic soil properties with spatio-temporal machine learning.
Using FORCE, the generation of bare soil maps is optimized for large areas and different time intervals. This can be challenging, as the soil properties across large scales are diverse, especially in regions with extreme conditions. New methods like spatial filters and dynamic thresholds are implemented to improve the representation of different soil regions and to reduce the noise of the soil reflectance composites. The results are stored in a bare soil data cube which is then used as a basis for soil monitoring. By combining this information with other environmental variables, such as climate and topology, the data cube approach can provide a basis to map soil dynamics over time. The bare soil cube is tested by predicting the SOC trends of cropland soils in Germany. Samples from the LUCAS campaigns, as well as from the Agricultural Soil Inventory in Germany (BZE-LW), are used to train and validate the models. The proposed approach has the potential to greatly improve the predictions of dynamic soil properties and to inform better management practices for sustainable land use, climate protection, and policymaking. Furthermore, the use of bare soil data cubes allows for efficient storage, retrieval, and analysis of large amounts of analysis-ready data, making it a practical and scalable approach for soil monitoring on a regional or global scale.
The use of synthetic aperture radar (SAR) data has become increasingly important in remote sensing for environmental monitoring. SAR data provides valuable information on surface characteristics and changes, such as land cover and land use change, soil condition, and vegetation growth, making it a powerful tool for various applications, including agriculture, forestry, and climate change studies. However, processing and integrating SAR data into analysis-ready formats can be complex and time-consuming, requiring specialized knowledge and tools.
In this contribution, we propose a software project called force-sar, which aims to integrate Sentinel-1 data into the Framework for Operational Radiometric Correction for Environmental monitoring (FORCE). FORCE is a widely used data cube framework for processing and analyzing optical remote sensing data and the integration of SAR data into FORCE increases its capabilities for large-scale, multi-modal analysis.
Force-sar automatically queries available Sentinel-1 Ground Range Detected (GRD) imagery covering the spatial and temporal dimensions of your area of interest. The scenes are then directly accessed from satellite data repositories on cloud environments like Creodias or the Copernicus Data and Exploitation Platform Germany (CODE-DE). When no connection to such repositories is available, the data can also be downloaded from data centers like the Alaskan Satellite Facility (ASF). The scenes are processed to radiometrically calibrated gamma-naught backscatter data using a pre-built but customizable ESA SNAP processing graph. After resampling, reprojecting, and tiling the data, they are ready for ingestion into a FORCE data cube.
The integration of Sentinel-1 data into FORCE allows for the creation of SAR data cubes with consistent radiometric and geometric properties, covering large regions and spanning multiple time periods. This enables users to perform multi-sensor and multi-temporal analyses like change detection, compositing, and classification and regression tasks for environmental monitoring at large scales, thereby supporting decision-making and policy evaluation in frameworks like the EUs Green Deal or the Common Agricultural Policy.
Force-sar is already used in the ongoing Mowing Detection Intercomparison Exercise (MODCiX), where more than ten teams compare their algorithms for grassland mowing detection on a consistent and harmonized remote sensing and reference data set. A consistent data cube holding optical and SAR data covering test regions in eight European countries was created and is used for the study.
Force-sar provides a streamlined and fully containerized workflow for preprocessing and integrating SAR data into an existing data cube framework without the need for the installation of any external tools or dependencies. This opens up new possibilities for utilizing SAR data in large-scale environmental monitoring applications, particularly when working in cloud environments.