Jasmin Fetzer
I am an environmental and soil scientist, having a broad education from Geoecology (Bachelor at the KIT, Karlsruhe), over Environmental Sciences (Master at the ETH Zürich), to Soil Science (PhD at the ETH Zürich the Federal Research Institute WSL, Birmensdorf). Now, I am working with our Startup Digit Soil on a portable device that can measure soil extracellular enzymatic activity. Within the AI4SoilHealth project we contribute to the section novel sensors and soil health parameters.
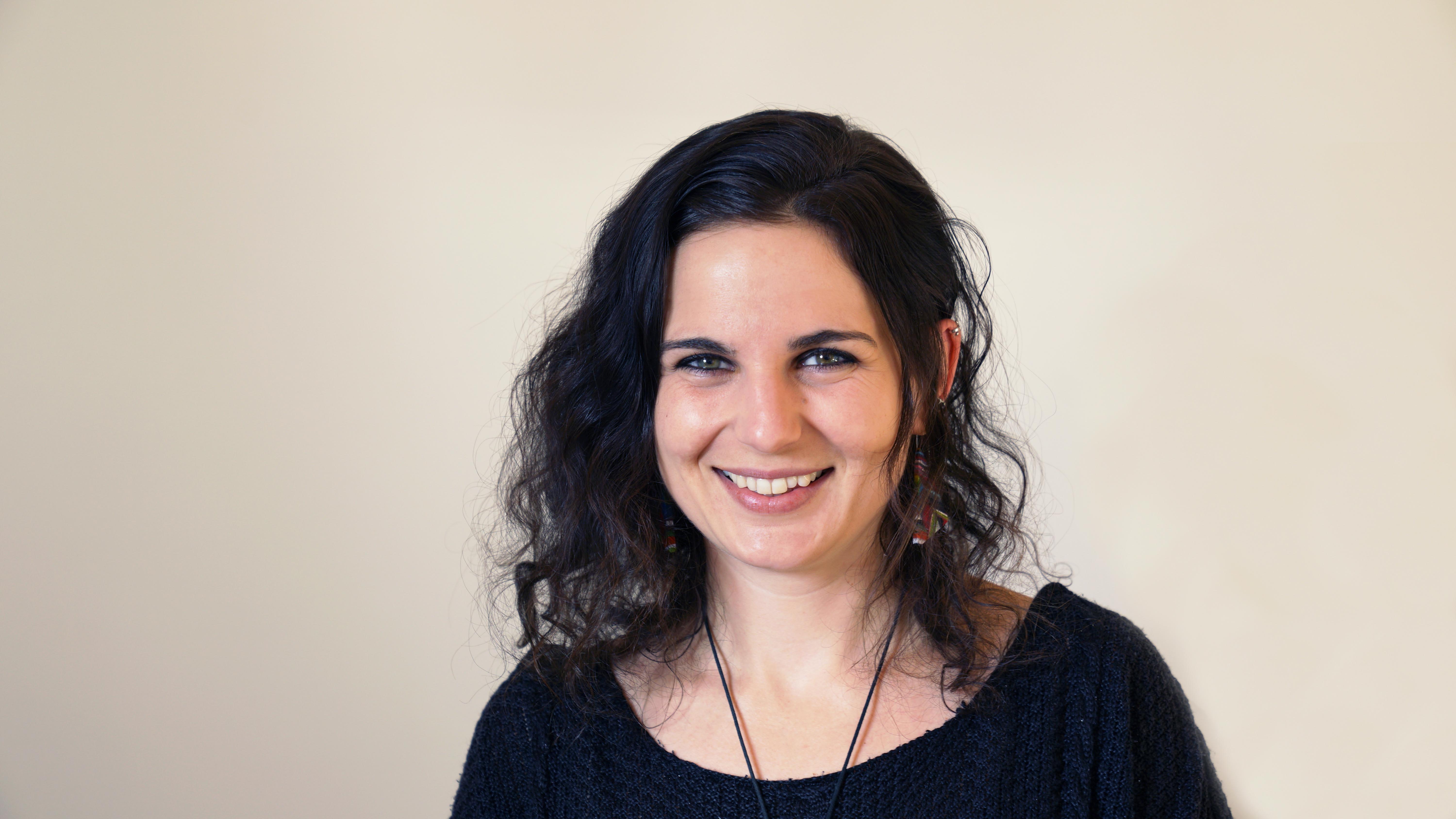
Sessions
Biological indicators play a critical role in assessing soil functions and overall soil health, yet they remain underrepresented compared to chemical and physical indicators. Soil health is strongly influenced by biological activity, which is essential for nutrient cycling, organic matter decomposition, and overall ecosystem productivity. Extracellular enzymatic activities (EEA), in particular, provide valuable insights into how soil biological activity responds to external factors such as management practices, climatic changes, or pollutants. However, traditional methods for measuring EEA typically require complex laboratory setups, which limits their application in real-time field assessments.
In this study, we introduce a novel, laboratory-independent soil enzyme activity reader (SEAR). SEAR utilizes an approach where soil enzymes react with fluorogenic substrates embedded in a transparent gel. Upon contact, the enzymes catalyze a reaction, producing fluorescent products that are detected on the opposite side of the gel. This enables a rapid and efficient assessment of multiple enzymatic activities, with the potential for analytical replicates and controls through the use of reaction plates with multiple gel compartments.
We validated SEAR by spiking sand samples with varying concentrations of different enzymes, thereby establishing operational limits for rate detection, precision, and substrate concentration ranges. Our results demonstrate that SEAR performs reliably across a wide range of soil types, including sandy to silty clay loam soils, acid forest soils (pH < 4), carbonate-containing agricultural soils, and soils with up to 18% organic carbon content. Furthermore, the device was tested under various environmental conditions, including soil moistures ranging from 2% to 173% of water holding capacity and temperatures from 6°C to 50°C, successfully demonstrating its versatility for field applications.
With SEAR, soil EEA measurements can be conducted quickly in the field, eliminating the need for laboratory access, sample storage, or pretreatment, which can alter results. The use of industrially manufactured reaction plates with strict specifications, combined with an automated data analysis pipeline, ensures standardized measurements without requiring specialized laboratory skills.
In conclusion, SEAR represents a significant advancement in soil biological assessment by enabling fast, accurate, and field-ready measurements of EEA. Its potential for standardization and ease of use positions it as a powerful tool for soil scientists and environmental managers to assess soil health and functionality in real time across diverse landscapes and conditions. SEAR will also enable ongoing monitoring of soil biological activity, supporting long-term studies and adaptive management practices for sustainable land use.
Policy demands for robust soil health monitoring are steadily growing. Given that soil biota are critical to the ecosystem services soils provide, biological properties are well-suited as relevant indicators, complementing physicochemical characteristics. However, biological properties are highly dynamic across spatial and temporal scales, which presents a challenge when using them for monitoring purposes.
As part of AI4SoilHealth project, we conducted a comprehensive soil health assessment in the experimental grasslands of NEIKER, where a rotational grazing system has been in place since 2013, compared to a free grazing system. Our objectives were to test innovative methods for measuring soil health and to analyze the temporal dynamics of soil biological properties in relation to climatic and pasture conditions.
Plant and soil samples were collected every three weeks from April to November 2024 at two depths (0-20 cm and 20-50 cm). A broad array of descriptors related to pasture quality, production, and soil physicochemical and biological properties were assessed. Novel methods tested and compared to conventional approaches included: (i) DigitSoil – a tool that measures enzymatic activity, providing real-time data on organic matter decomposition and other key biological processes; (ii) microBIOMETER – a portable kit measuring microbial biomass and the fungi-to-bacteria ratio; (iii) Slakes – assessing aggregate stability through a mobile app; (iv) eDNA and eRNA metabarcoding of 16S rRNA and ITS, to differentiate total and active prokaryotic and fungal communities; (v) remote sensing from Planet to provide data on vegetation growth and greenness.
The novel diagnostic tools provided cost-effective and high quality soil health assessments. Nevertheless, preliminary results suggest that differences in soil biological properties were more pronounced across soil depths and over time than between grazing types. Therefore, their spatial and temporal variability must be considered when designing a soil health monitoring program.
Soils are increasingly rediscovered as a vital resource that underpins many natural and societal services. Over more than half a century, agricultural mechanization and a singular focus on plant production, supported by chemical fertilizers, have led to widespread soil degradation. This reductionistic perspective has relied on soil observations focused on physico-chemical properties; properties that can be boosted by chemical additions but ignore the biological and ecological status and functions of the soil. Recognizing the importance of natural soil processes, which have evolved and been fine-tuned over billions of years, a new set of indicators for describing soil health beyond the physico-chemical properties is required. These indicators should preferably be observable and analyzable by farmers, advisors, extension workers and other citizen scientists. Methods that directly or indirectly capture the biological and ecological functions include, for instance i) environmental DNA (eDNA) metabarcoding to characterize the diversity and composition of soil microbial communities, ii) activity rates of key enzymes involved in the main biogeochemical cycles, iii) the ratio of soil fungi to bacteria, an indicator of the extent of disturbance in soil ecosystems, iv) aggregate stability, which is important for soil erosion resistance, and water and nutrient holding capacity, and v) water infiltration capacity as a key measure of the soil water absorption, holding and release potentials. While eDNA requires specialist laboratories and databases, the other methods are currently available for “Do-It-Yourself” (DIY) testing. In this study, as part of the EU-funded project AI4SoilHealth (https://ai4soilhealth.eu) we sampled soils in Greece, Sweden, Finland, Croatia and Denmark. We applied the outlined methods alongside traditional wet chemistry analysis of properties such as carbon, pH and electrical conductivity, and the particle size distribution. These properties were also estimated by leveraging their correlations with diffuse reflectance Near InfraRed (NIR) spectra and applying machine learning models. We are testing both the robustness of the novel methods and their interdependence with more traditional physico-chemical properties and soil spectroscopy. We hypothesize that there is a significant positive correlation between novel indicators (e.g. eDNA richness is correlated to enzymatic activity, which is correlated to aggregate stability, which in turn is correlated to infiltration capacity) and that high scores of the biological and ecological properties are correlated with, for instance, soil carbon content. This study explores the potential of these novel methods for more holistic understanding of soil health.
Most soil properties are continuously varying over different scales in both space and time. An analysis of field sampled soil is the most accurate method for estimating the spatial distribution of soil properties and health indicators at field scale. The field scale spatiotemporal variation in properties, however, requires an effective, well selected and unbiased probability distribution based sampling framework leveraging the in-situ variability of the relevant environmental covariates. Covariates that can be used for modeling the soil properties over the entire study area. Typically such covariates are represented at spatial rasters derived from e.g. topographic data and satellite imagery. In this work, we introduce the probability based balanced stratified sampling algorithm compatible with the proposed European Soil Monitoring law. The algorithm distributes doubly balanced sampling locations over both geographical and feature space constrained by a maximum allowed error – in our case the coefficients of variation. The geographical feature space input data are selected covariates from the Soil Health Data Cube (https://shdc.ai4soilhealth.eu/). We target the covariates that reflect the distribution of soil health indicators tested or developed by the EU funded project AI4SoilHealth (https://ai4soilhealth.eu). To begin this process, a Bethel-inspired optimization approach is applied to stratify the study areas. The strata are then used for computing the approximately equal inclusion probabilities for all units and the number of samples for each strata allocated based on the available auxiliary information. The second stage of the process involves doubly balancing the algorithm, with the aim to select the optimal sampling locations over geographical and feature space with respect to the stratification. In our study, we compare the algorithm with 1) simple random sampling, 2) feature space coverage sampling and, 3) the EU wide Land Use and Cover Area frame Survey (LUCAS) algorithm using legacy data. The advantages of the novel algorithm are demonstrated in the optimized number of samples while preserving the accuracy of target estimates and mapping accuracy. In the first experiment, we subsample the legacy random grid samplings. In the second (numerical) experiment, we use the soil property maps of the Soil Health Data Cube as observed values to design new optimized sampling networks with no gridded location constraints.