Annett Wania
Annett Wania holds a PhD in Geography and has 21 years of experience in using geospatial and Earth Observation data for the analysis of the impact of human activities. After obtaining her PhD in Geography from the University of Strasbourg, France, she has been working at the European Commission’s Joint Research Centre for 13 years on applications in the environmental and agricultural domain as well as applications on urban environments and disaster management. During the last six years at JRC she was working on satellite-based mapping for disaster management under the Copernicus Emergency Management Service. During her time at the JRC she has transitioned from conducting technical work to managing scientific and technical projects and teams. Since January 2021 she is working at Planet Labs in the Earth Observation Lab, where she is managing a team of six engineers and data scientists which is implementing a number of research and development projects aiming at testing and further developing Planet’s image products for applications in the environmental and agricultural domain (crop classification, phenology, environmental impact of mining activities). In addition to traditional remote sensing methods, the team’s focus is on experimenting with innovative machine learning techniques to extract information from Planet’s high-cadence imagery and multi-modal datasets and define solutions for customers, which help them build new applications based on Planet data.
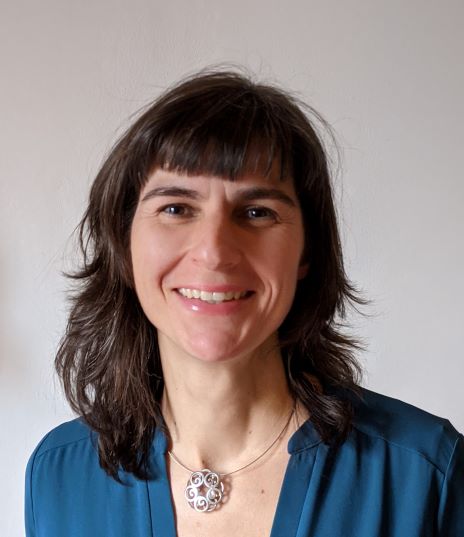
Sessions
Compaction reduces the infiltration capacity of the soil surface and may result in water ponding during heavy rainfall events. The same ponding effect is expected for soils with low organic content, limiting the formation of structural pores with high drainage capacity. The resulting presence of ponding water generates run-off and anaerobic conditions in the topsoil, increasing erosion rate and reducing the biological activity and productivity. The longer the duration of a rainfall event, the lower the infiltration capacity of the soil and the higher the risk that the infiltration capacity becomes limiting, defining the ‘ponding time’ as the time of onset of water accumulation at the surface. The ponding time is a comprehensive soil physical property, integrating aspects of soil water retention, hydraulic conductivity, and initial water content. The lower the ponding time for a certain precipitation rate, the more frequent is the expected occurrence of water ponding at the surface. In this study, we apply an analytical expression for ponding time to compute it at the Pan-European scale. Using maps of basic soil information (soil texture, organic carbon, and bulk density), we can estimate values of ponding time and thus the frequency of ponding (and run-off) by infiltration excess. This map provides a reference for the quantification of soil health by reducing ponding time by compaction and loss of organic carbon (‘unhealthy’) and increasing ponding time by structure formation (‘healthy’). Applying pedotransfer functions linking soil hydraulic properties with basic soil information and land use, we provide a sensitivity map to estimate the change in ponding time statistics by modifying land management. The generated maps have a spatial resolution of 1 km and allow a comparison with national statistics of hydromorphic constraints, revealing the relationship between infiltration excess and saturation excess. To apply ponding maps in soil health assessment and land use management, they must be developed at higher resolution. We will show the potential of providing ponding maps at higher resolution (30 m, Soil Health Data Cube for Europe) and linking them to remote sensing observations of ponding using satellite data.
The advent of modern satellite constellations–combined with significant advances in computational technology and resources–enables the systematic mapping of agricultural areas in high spatiotemporal resolution and through this provides a foundational building-block for the study and monitoring of soil health. In this context, parts of the Earth Observation and Machine Learning community have placed a growing focus on the development of capabilities to remotely identify crop types growing on agricultural cropland. These capabilities can support soil health monitoring efforts by providing systematic insights into crop rotations and cropping practices that affect soil health (e.g. cover crops). End users of these capabilities desire high accuracy across large areas with diverse agro-environmental conditions, as early in the season as possible.
Whilst recent efforts have typically shown strong performance on datasets that express limited spatial and/or temporal variability, the community is yet to explore performance across an adequate number of years at the pan-European scale to assess robustness both to the spatial variations between environmental & political zones and to the full range of variations in interannual weather patterns. To do so, there is still a need for datasets that provide sufficient spatial and temporal depth.
In Europe, the public release of historical LPIS and GSAA datasets by EU member states at either regional or national scales has made high quality crop type ground-truth data more available than ever before. The RapidCrops dataset combines these datasets across a range of countries and multiple years to provide a deep spatio-temporal stack of crop type ground-truth data; enabling assessments of generalization across both space and time. The dataset leverages harmonization standards developed under the EuroCrops initiative to standardize data from different countries. It adopts & extends the fiboa (https://fiboa.org) field boundaries data standard to make parcel boundaries and crop type labels available in an open, interoperable format. Finally, the dataset provides additional usability metrics for each parcel to help users identify and access the data most appropriate to their context, quickly.
Boermarke-Zeijen is a 350-year old farmers collective in the North of the Netherlands. The farmer collective
owns 1200 hectares of land and consists of five dairy farms, four arable farms, two mixed farms, and one chicken
farm. The farmers are developing economically and ecologically sustainable farming practices to strengthen soil
fertility and biodiversity at company and regional scale. The soils are sandy with peat patches, which is
representative of the soils of the Netherlands. The farmers producing agricultural crops face subsoil compaction
and soil crusting, leading to decreased infiltration capacity. Some of the dairy farms face phosphate deficiencies.
As part of AI4SoilHealth project, Planet Labs is teaming up with the farmer collective and the regional water
board Noorderzijlvest, to correlate in-situ measurements with Planet’s satellite data products to demonstrate its
value for monitoring and decision making. The farmers have a specific interest in monitoring nutrient fluxes, soil
moisture, land surface temperature and biomass conditions, specifically for the sustainable application of
fertilizer.
The in-situ monitoring network collects location-specific, real-time information about the weather outlook, the
level of surface water and groundwater, the moisture content of the topsoil and the water quality. Legacy data
includes in-situ samples and soil profiles covering a range of soil parameters. A field sampling campaign is
planned in April 2025 to collect the AI4SoilHealth baseline indicators (texture, SOC, pH, CEC, nutrients, density)
and additional indicators (e.g. enzymatic activity, eDNA, Macrofauna, NIR-spectroscopy).
We have used Planet’s high spatial and temporal resolution satellite data [Planet Product] to monitor and
characterize the landscape of Boermarke Zijen, by looking at Vegetation health [PlanetScope, Crop Biomass];
Water balance [Soil Water Content]; Environmental stresses [Land Surface Temperature]; Soil health [Tanager,
Sentinel, PlanetScope] and Above ground carbon [Forest Carbon, Crop Biomass]. With the recent launch of
Planet’s Hyperspectral Mission Tanager, the pilot at Boermarken Zijen will also facilitate investigations into the
feasibility of soil property estimation using spaceborne hyperspectral sensors. Using our satellite data products
in combination with the in-situ sample data, will evaluate the water regime and the potential and effects of
year-round greenery to address some of the challenges that the farmers at Boermarke Zijen are facing.