Xuemeng Tian
Hello! I'm a PhD student with OpenGeoHub and Wageningen University, focusing on spatial-temporal modeling of soil organic carbon using machine learning techniques. My daily tasks involve organizing (messy) soil data, processing (a lot! of) earth observation data, developing and testing (weird) algorithms, as well as drinking coffee (quarreling or gossiping) with (lovely) colleagues. Glad to meet you and look forward to discussing soil science and everything else!
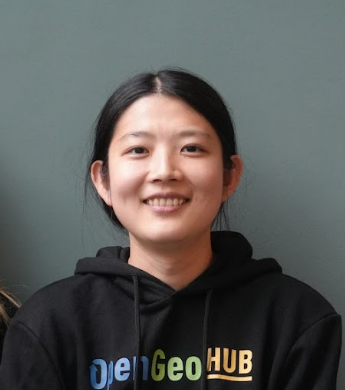
Sessions
This work presents a comprehensive framework for soil organic carbon density (SOCD) (kg/m3) modeling and mapping, based on spatiotemporal Random Forest (RF) and Quantile Regression Forests (QRF). 22,428 SOCD measurements and a wide range of covariate layers—particularly the 30m Landsat-based spectral indices were used to fit models and produce 30m SOCD maps for the entire EU at four-year intervals from 2000 to 2022 and for four soil depth intervals (0--20cm, 20--50cm, 50--100cm, and 100--200cm) each accompanied by per-pixel 95% probability prediction intervals (PI, between P0.025 and P0.975). The results of model evaluation indicate consistent accuracy of the predictions: based on both 5--fold spatial cross-validation with model refitting (MAE = 8.64 kg/m3, MedAE = 4.31 kg/m3, MAPE = 0.54 kg/m3 and bias = -2.95 kg/m3), and on independent testing (MAE = 7.73 kg/m3, MedAE = 3.54 kg/m3, MAPE = 0.45 kg/m3, and bias = -3.04 kg/m3), with both R2 values exceeding 0.7 and concordance correlation coefficients (CCC) greater than 0.8. Validation of PI estimation confirmed that PIs effectively capture uncertainty intervals, although with reduced accuracy for higher SOCD values. Exploratory analysis using Shapley values identified soil depth as the most important feature, with vegetation (Landsat biophysical indices) and long-term bio-climate features as the two main contributing feature groups. Although the uncertainty of the prediction per pixel is significant, further spatial aggregation has been shown to reduce the uncertainty by about 70%. Suggested uses of the data include: (1) time-series / trend analysis to detect potential land degradation hotspots, (2) optimization of sampling designs based on prediction uncertainty, and (3) prediction of future soil carbon potential by extrapolating models under different land use / climate scenarios. The data and code used are publicly available under an open license from https://doi.org/10.5281/zenodo.13754344 and https://github.com/AI4SoilHealth/SoilHealthDataCube/.
Automated Machine Learning (AutoML) is today of interest to many production teams looking for faster and more robust data production (see e.g. https://youtu.be/aiM_9r5strw). Large-scale soil property mapping is challenging due to the significant computational resources required and the extensive human effort needed to locate, harmonize, and prepare data (including measurements and covariates) that align with the target spatial and temporal modeling scales. To address these challenges, we developed a modular framework EO-soilmapper that automates the workflow as much as possible (read more in: https://doi.org/10.21203/rs.3.rs-5128244/v1). Our framework introduces three main components: (1) ready-to-use EU-scale covariate layers—a comprehensive and consistent set of covariates along with the process for their preparation, (2) a harmonized EU soil property point database that integrates and quality-controls soil point data from multiple sources, and (3) “scikit-map” (https://github.com/openlandmap/scikit-map) a Python package that enables a highly automated execution pipeline, minimizing manual operation. Scikit-map supports spatial-temporal point overlay, spatial machine learning, spatial-temporal mapping, parallelized processing, etc. Together, these components streamline workflows, reduce manual input, and ensure consistency across large datasets. These tools and resources can be readily adapted for other machine learning applications in environmental modeling and mapping, further supporting the open-source soil data communities.