Mahsa Nakhostinrouhi
I am a researcher working on soil contaminants. I deal with field and spectrometry data measured from soil samples. I am skilful at applying a variety of artificial intelligent methods and tools, including machine learning, explainable machine learning, and python programming in this field of work. Furthermore, I am proficient at using remote sensing imagery to analyse and get information, specially from soil. To wrap up, I combine soil spectrometry, remote sensing, and artificial intelligence to advance our understanding of soil health.
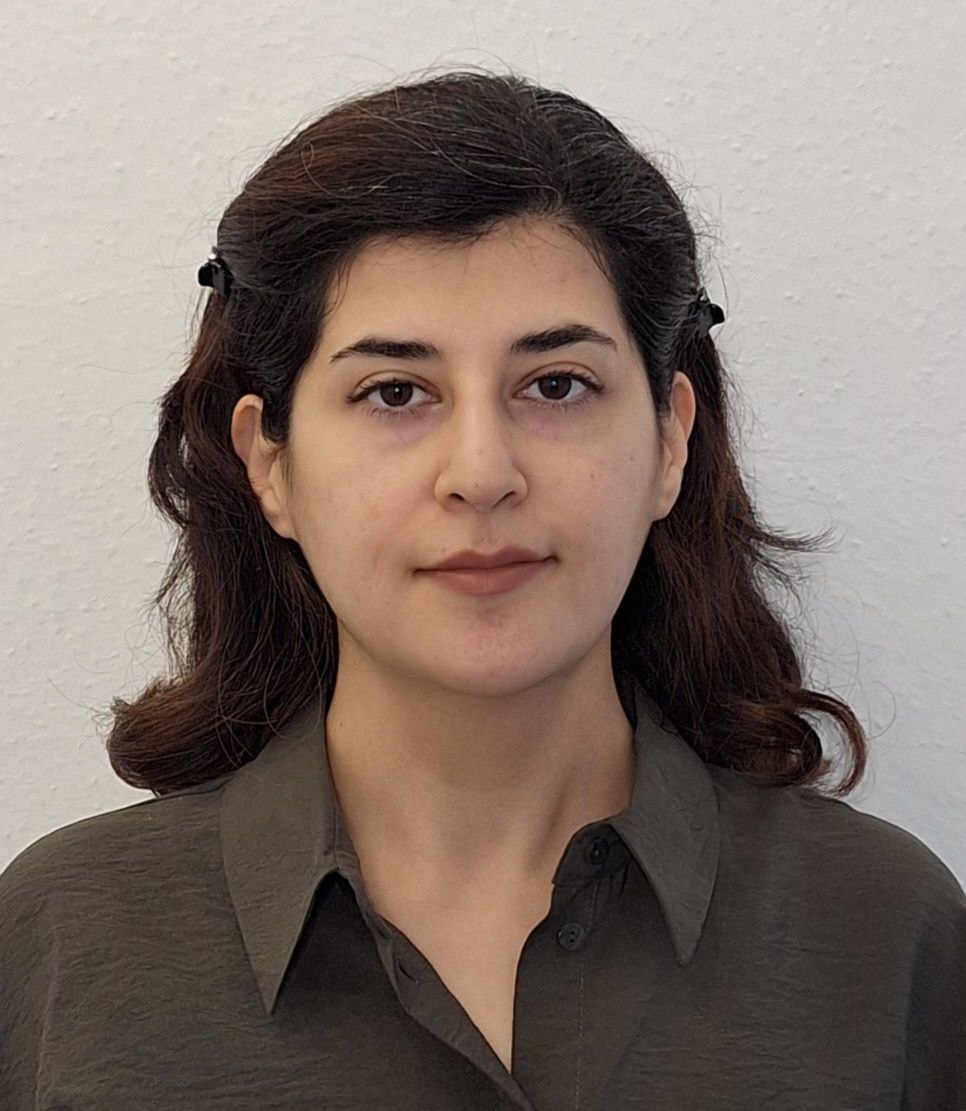
Sessions
There is a strong need to investigate soil health due to its big role in environmental and human health. In terms of this issue, there could be some elements to be explored. Heavy metals are important among these elements since their increase from specific thresholds would lead to soil pollution and ultimately impact human health. One of the heavy metals with a high influence on soil health is nickel (Ni). It is becoming more important in urban areas because of the high potential for growth through heavy transportation. In this study, 89 surface soil samples (0-20 cm) were collected from traffic areas nearby streets in Berlin, Germany in 2018. Then they were sieved and dried in the laboratory. Next, the amount of Ni and spectra in VNIR-SWIR bands were measured in the laboratory for each sample with an ASD FieldSpec5 spectroradiometer under controlled illumination conditions. The spectral reflectance data were transformed to absorbance values as a preprocessing method. In the next step, the data were split into two groups: training (80%) and test (20%) data. The first group was fed into random forest algorithm to develop a model for predicting Ni. Then the test set was utilized to validate the predicting ability of the model. Regarding the hyperparameter tuning of the model, GridSearchCV method was applied to find the best values for n_estimators, and max_depth. What is not considered in most of the similar studies is interpretability of a machine learning model produced leaving that in a black-box situation. Hence, in this study two explainable machine learning (eXML) methods, including MDI (Mean Decrease in Impurity), and permutation were applied to determine VNIR-SWIR bands with higher importance in the modeling and predicting of Ni. When it comes to the results, the validation of the random forest model yielded an R2 of 0.74 and an RMSE of 2.01. This proves that the models’ predictions align well with the actual Ni values. Furthermore, the results achieved from MDI method specified the wavelengths 2418nm, 2127nm, and 2147nm (short wave infrared) as three most important features in the random forest model generated for Ni. On the other hand, the features 2127nm, 401nm, and 2147nm were determined the most important features by permutation method for the model trained. This information could be very useful in using remote sensing sensors in Ni prediction in large scales specifically in an urban area.