Sarem Norouzi
As an Agroecology PhD fellow at Aarhus University, I work on physics-based and physics-informed machine learning approaches for soil property mapping in the AI4SoilHealth project. My work also involves developing soil spectral models and using machine learning for the prediction of soil hydraulic parameters and texture at larger scales. By integrating existing knowledge into model training, I aim to enhance spatial accuracy and effectively handle sparse datasets.
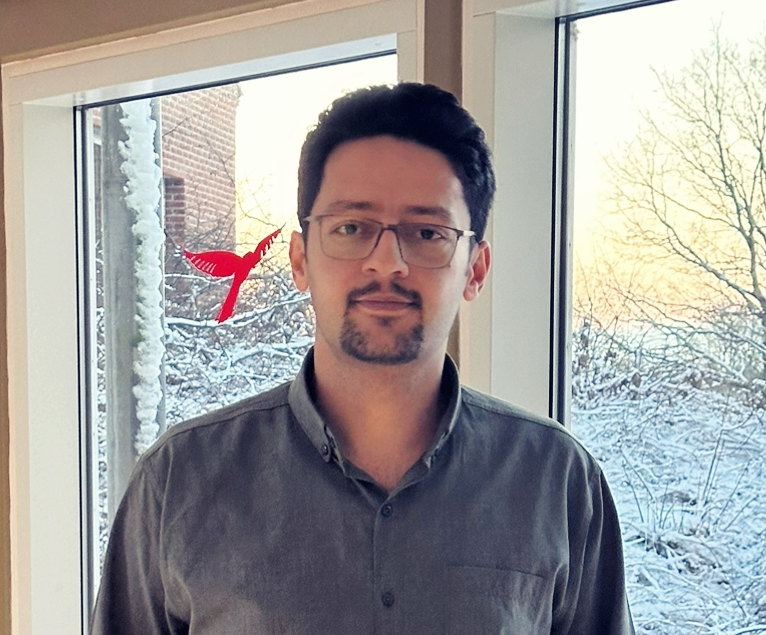
Sessions
Over the past two decades, machine learning (ML) methods have been widely applied in soil science to estimate various soil properties. Despite its successes, traditional ML methods encounter several limitations, such as high data requirements, poor generalization to new scenarios, and challenges in ensuring physically consistent predictions beyond the range of their training data. To overcome these limitations, we propose the use of physics-informed machine learning (PIML) methods, which integrate physical laws directly into the learning process to enhance model robustness and generalizability, particularly in data-limited scenarios. We demonstrate the capabilities of PIML by developing two PIML models for the estimation of two fundamental soil properties related to soil health, soil particle size distribution (PSD) and soil water retention curves (SWRC), using measured spectral reflectance data (400–2500 nm). Unlike conventional approaches, these PIML models are designed to learn non-specific continuous forms of SWRC and PSD by effectively incorporating both observational data and physical laws during training. This novel approach offers capabilities beyond those achievable with traditional methods. Specifically, the PIML model for PSD can seamlessly integrate both complete and incomplete measurements from diverse soil classification systems without requiring harmonization through interpolation of inputs, and is able to make predictions in any soil classification system. This makes the proposed approach particularly suitable for modeling PSD across datasets collected from various countries with different soil classification systems, such as at a pan-European scale. Additionally, The PIML model for SWRC can effectively handle samples with sparse or incomplete measurements, making it well-suited for SWRC datasets that often contain missing sections of the curve or limited data points. The proposed PIML approach provides a fast and cost-efficient method for monitoring fundamental soil properties to evaluate key soil health indicators. It can also be adapted for proximal sensing and digital mapping of other soil health-related properties.