Thomas Gumbricht
I divide my time between being a researcher at Stockholm University and working with a start-up company developing environmental sensors for citizen scientists. My research focuses on combining field data and satellite images for understanding and modelling landscape health and processes. My special interest is tropical wetlands and their functions.
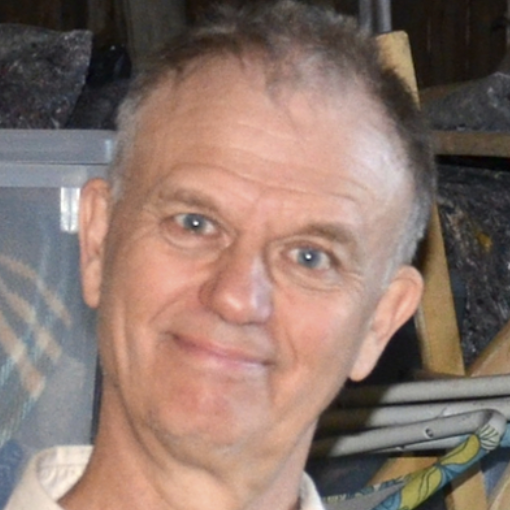
Sessions
Most soil properties are continuously varying over different scales in both space and time. An analysis of field sampled soil is the most accurate method for estimating the spatial distribution of soil properties and health indicators at field scale. The field scale spatiotemporal variation in properties, however, requires an effective, well selected and unbiased probability distribution based sampling framework leveraging the in-situ variability of the relevant environmental covariates. Covariates that can be used for modeling the soil properties over the entire study area. Typically such covariates are represented at spatial rasters derived from e.g. topographic data and satellite imagery. In this work, we introduce the probability based balanced stratified sampling algorithm compatible with the proposed European Soil Monitoring law. The algorithm distributes doubly balanced sampling locations over both geographical and feature space constrained by a maximum allowed error – in our case the coefficients of variation. The geographical feature space input data are selected covariates from the Soil Health Data Cube (https://shdc.ai4soilhealth.eu/). We target the covariates that reflect the distribution of soil health indicators tested or developed by the EU funded project AI4SoilHealth (https://ai4soilhealth.eu). To begin this process, a Bethel-inspired optimization approach is applied to stratify the study areas. The strata are then used for computing the approximately equal inclusion probabilities for all units and the number of samples for each strata allocated based on the available auxiliary information. The second stage of the process involves doubly balancing the algorithm, with the aim to select the optimal sampling locations over geographical and feature space with respect to the stratification. In our study, we compare the algorithm with 1) simple random sampling, 2) feature space coverage sampling and, 3) the EU wide Land Use and Cover Area frame Survey (LUCAS) algorithm using legacy data. The advantages of the novel algorithm are demonstrated in the optimized number of samples while preserving the accuracy of target estimates and mapping accuracy. In the first experiment, we subsample the legacy random grid samplings. In the second (numerical) experiment, we use the soil property maps of the Soil Health Data Cube as observed values to design new optimized sampling networks with no gridded location constraints.