Hamidreza Mosaffa
I'm a Postdoctoral Researcher at the National Research Council of Italy (IRPI-CNR), working with Prof. Luca Brocca. I'm interested in developing machine learning tools to enhance hydrological monitoring and modeling. This work aims to improve our ability to mitigate natural hazards. My current research is focused on high-resolution satellite products with deep learning algorithm and create hybrid physics-aware models.
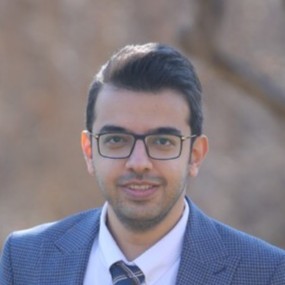
Sessions
Accurate and timely flood risk assessment is paramount for effective disaster mitigation and preparedness. Traditional flood susceptibility maps (FSMs) often fall short by providing static representations, failing to capture the dynamic nature of flood risk in a changing climate. This study presents a novel dynamic FSM framework that integrates high-resolution climate data and temporal analysis to address these limitations. Developed within the context of the Open-Earth-Monitor Cyberinfrastructure (OEMC) project, our methodology offers a significant advancement in flood risk modeling.
To generate dynamic, high-resolution (1 km) FSMs for the Mediterranean region, we utilized the Random Forest algorithm. These maps uniquely adapt to varying seasonal conditions, precipitation intensities, and post-drought scenarios. Our model's adaptability stems from its training on a comprehensive dataset that combines flood and non-flood locations from the Copernicus Emergency Management Service (EMS) and the Global Flood Database v1. Additionally, we incorporated crucial factors influencing flood events, including elevation, slope, land cover, drainage density, soil moisture, and precipitation. Model evaluation employed cross-validation techniques utilizing both training and testing datasets. This comprehensive assessment confirmed the superior performance of the Random Forest model, solidifying its effectiveness as a robust tool for flood susceptibility mapping.