Gebeyehu A. Zeleke
Gebeyehu Abebe (Ph.D.), an assistant professor at Debre Berhan University in Ethiopia, specializes in remote sensing. His research interests span crop growth monitoring, SAR image processing, data fusion, machine learning algorithms, and crop modeling. Currently, he’s conducting research as part of the 2023 African Research Fellow program at ESA/ESRIN in Frascati, Italy.
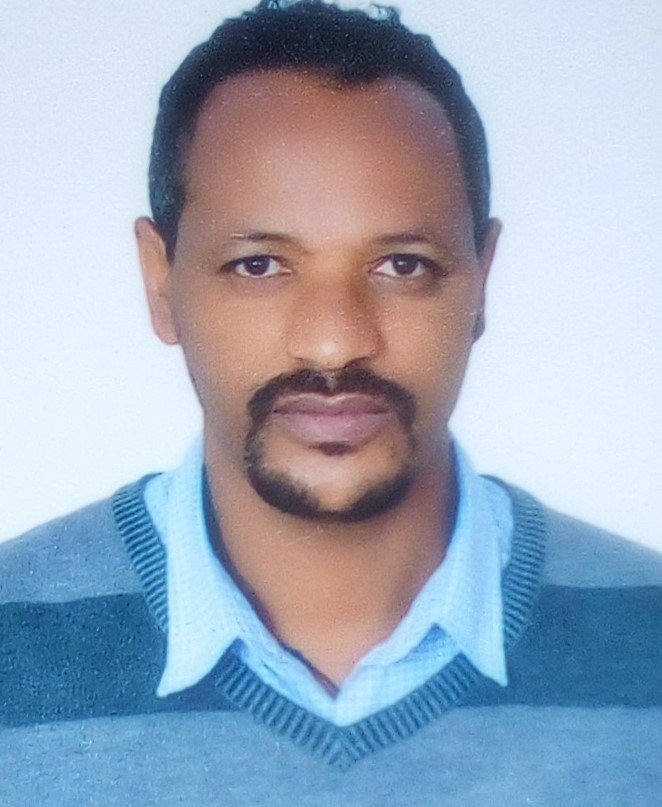
Sessions
Assimilating Leaf Area Index and Soil Moisture from Optical and SAR Data into the WOFOST Model to Improve Maize (Zea mays L.) Yield Estimation
Gebeyehu Abebe 1,2, Odunayo David Adeniyi1,3, Amazirh Abdelhakim1,4, Zoltan Szantoi1
1European Space Agency (ESA)/ESRIN, Frascati RM 00044, Italy
2Department of Natural Resources Management, Debre Berhan University, Debre Berhan, Ethiopia.
3Department of Earth and Environmental sciences, University of Pavia, Italy, Via Ferrata 1, Pavia, 27100, Italy.
4Centre for Remote Sensing Applications (CRSA), Mohammed VI Polytechnic University (UM6P), Hay My Rachid, Ben Guerir 43150, Morocco
Abstract
Crop Simulation Models (CSM) are commonly used to estimate crop yield at a local scale. Meanwhile, Remote Sensing (RS) data provides valuable information on crop parameters like soil moisture and leaf area index (LAI) across different spatial scales. Data Assimilation (DA) is a powerful technique that combines CSM and RS data from satellite imagery to enhance simulated crop state variables and model outputs, such as total biomass and yield. In this study, we aimed to implement a joint assimilation strategy for LAI and soil moisture data in the WOFOST model. The goal was to simulate rainfed grain maize yield at the field scale and evaluate its performance at both the field and administrative zone levels. The Ensemble Kalman Filter (EnKF) algorithm was applied to achieve this integration. The LAI and soil moisture data were sourced from Sentinel 3 and Soil Moisture Active Passive (SMAP) L3 Radiometer Global Daily 9 km Soil Moisture, respectively. The study tested various assimilation scenarios, including deterministic modeling, independent assimilation of LAI from Sentinel 3, independent assimilation of soil moisture from SMAP, and joint assimilation of both LAI and soil moisture data. Ongoing validation involves comparing the simulated grain maize yield with field observations and independent grain maize statistics data in the major maize-growing administrative zones of western and southwestern Ethiopia. The expected outcomes include improved accuracy in grain maize yield predictions at the field scale and enhanced crop monitoring and forecasting at local and national levels.
Keywords: Data assimilation; EnKF; LAI; soil moisture; WOFOST; grain maize yield